Join the Machine Learning in Medical Imaging Consortium (MaLMIC) for an opportunity to network
Machine Learning in Computational Pathology
Friday, May 26, 2023
3:00 to 4:30 p.m. ET
For the May forum on machine learning in computational pathology, the first presentation by April Khademi, a researcher, will be about the past, present and future of digital pathology and AI. The second presentation by Phedias Diamandis, a pathologist, will be about approaches to solve implementation challenges of AI in the real world. The talks will be followed by discussion focused on lessons learned, opportunities to collaborate, and sharing of resources.
Interested in joining? Please contact us.
Machine Learning in Computational Pathology
Friday, May 26, 2023
3:00 to 4:30 p.m. ET
For the May forum on machine learning in computational pathology, the first presentation by April Khademi, a researcher, will be about the past, present and future of digital pathology and AI. The second presentation by Phedias Diamandis, a pathologist, will be about approaches to solve implementation challenges of AI in the real world. The talks will be followed by discussion focused on lessons learned, opportunities to collaborate, and sharing of resources.
Interested in joining? Please contact us.
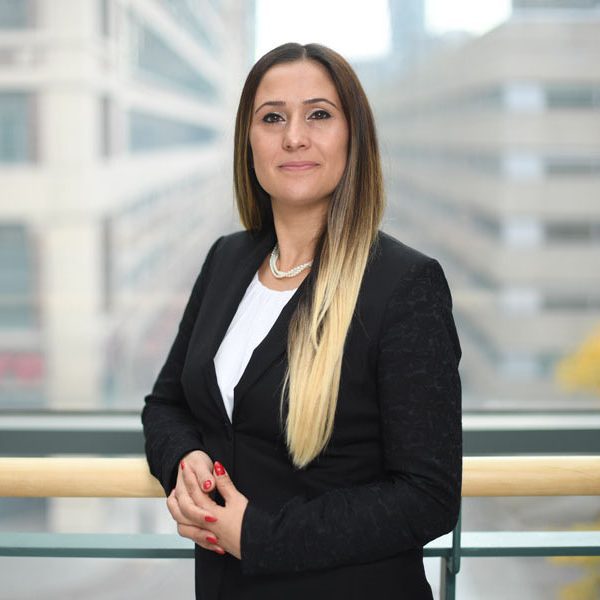
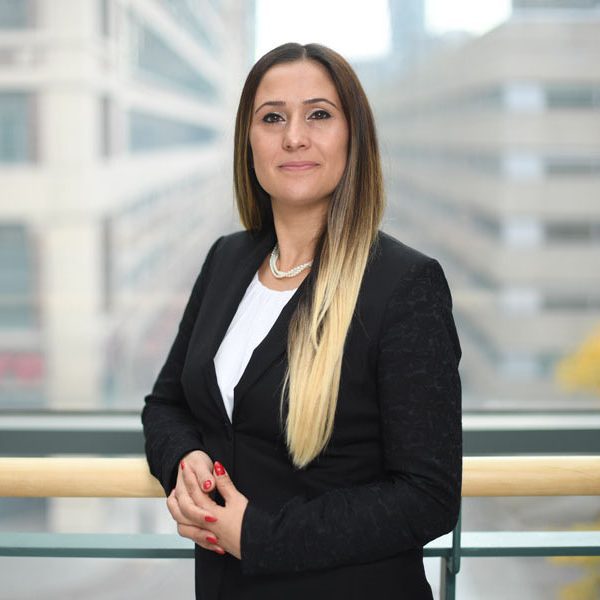
Digital Pathology + AI: Past, Present and Future
April Khademi, PhD
Assistant Professor, Metropolitan Toronto University
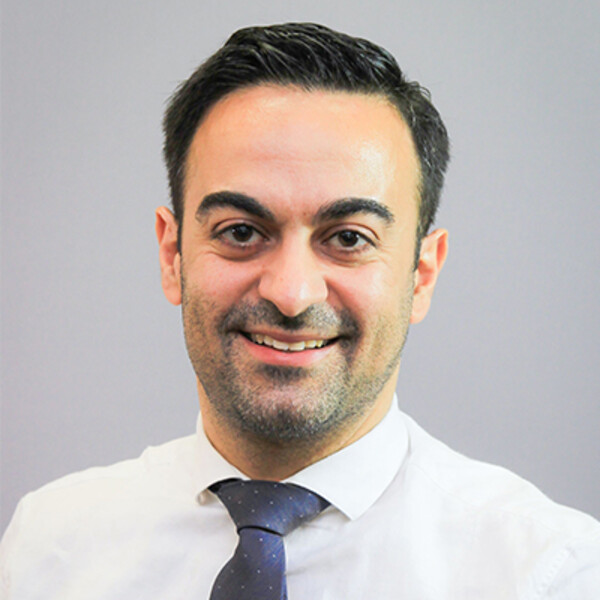
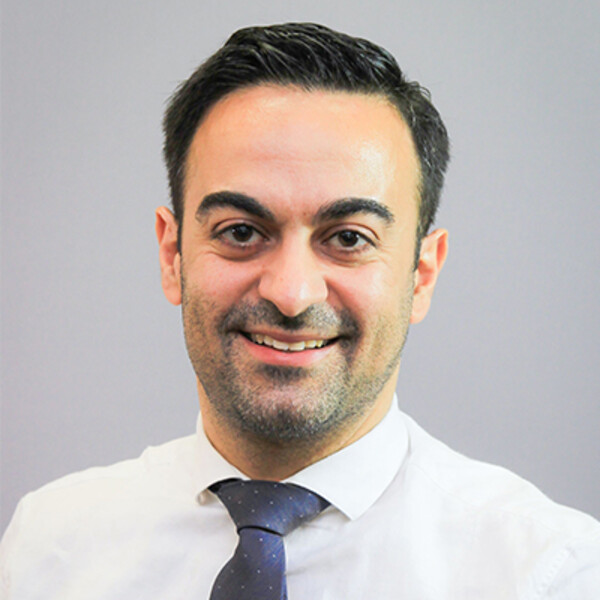
Living on the Edge: Challenges to implementing AI in the real world
Phedias Diamandis, MD, PhD
Associate Professor, University of Toronto
Deep learning has emerged as a powerful approach for automating and objectifying numerous manual and qualitative image-based interpretive tasks. Notwithstanding accumulating breakthrough proofs-of-concepts, the existence of superfluous “edge cases” and context-specific applications in the real world has limited wide-spread adoption. In this talk, I use various examples of everyday decision-making tasks in pathology to highlight challenges with real-world applications of AI. I highlight similar challenges and solutions in cataloging human history and propose the need for similar crowd-sourcing approaches to overcome existing barriers in the implementation of AI in the real world