Join the Machine Learning in Medical Imaging Consortium (MaLMIC) for an opportunity to network on machine learning
Machine learning for molecular and cellular imaging
Friday, April 22, 2022
3:30 to 5:30 p.m. EDT
Hartland Jackson will talk about machine learning applied to imaging mass cytometry. Parvin Mousavi will present her work on mass spectrometry applied to real-time intraoperative tumour margin detection. David Andrews will talk about machine learning in high throughput single-cell imaging. The talks will be followed by discussion focused on lessons learned, opportunities to collaborate and sharing of resources.
Interested in joining? Please contact us.
Machine learning for molecular and cellular imaging
Friday, April 22, 2022
3:30 to 5:30 p.m. EST
Hartland Jackson will talk about machine learning applied to imaging mass cytometry. Parvin Mousavi will present her work on mass spectrometry applied to real-time intraoperative tumour margin detection. David Andrews will talk about machine learning in high throughput single-cell imaging. The talks will be followed by discussion focused on lessons learned, opportunities to collaborate and sharing of resources.
Interested in joining? Please contact us.
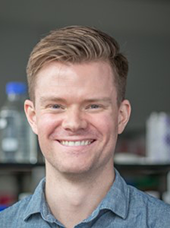
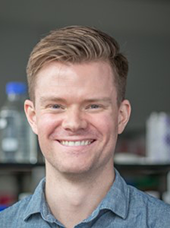
Highly multiplexed histopathology imaging to study the cellular organization of tissue
Hartland Jackson, PhD
Investigator, Lunenfeld-Tanenbaum Research Institute
Talk summary: Hartland will discuss the application of machine learning methods to imaging mass cytometry and other highly multiplexed imaging methods to study the spatially resolved single cell content of human tissues, and how multi-cellular features can be used as potential biomarkers and to understand cell communication networks which drive disease progression.
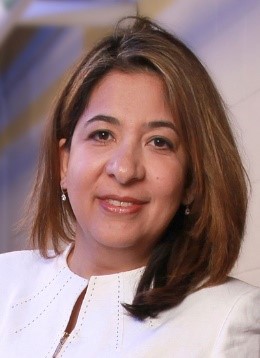
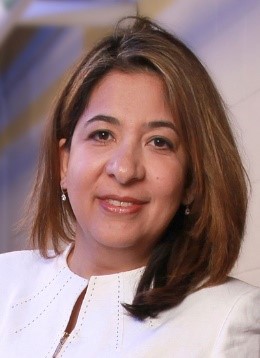
Assessment of resection margins in surgical oncology using mass spectrometry: interpretable learning models
Parvin Mousavi, PhD
Professor, School of Computing, Queen’s University
Talk summary: Incomplete tumor resections lead to presence of cancer at the margins, demanding subsequent revision surgery and poor outcomes for patients. Intraoperative evaluation of tissue pathology, including the surgical margins, can help decrease the burden of repeat surgeries on patients and healthcare systems. This talk will review some of our recent efforts in using intraoperative mass spectrometry and deep learning for assessment of cancer at resection margins. Specifically, it will focus on interpretable learning models that are robust to the noise in the data and the uncertainty of the tissue labels.